
MCA (Measure Cognition and Action)
It is a tool developed together with our partners COGNITIVE-MOTOR PERFORMANCE SPA., which applies evaluation and training methods to optimize the cognitive-motor skills of athletes.

Intelligent Sensing System for the Agro-Fruit Industry – (SIAG)
Wildsense Ltda. develops an IOT sensing system to assist in data extraction and relevant information in critical processes related to agricultural cultivation, specifically in fruit production.

Automated Subsea Robotics for High Seas Salmon Farming (R-SASA)
Inspection processes in offshore salmon farming cages currently have low technological sophistication, accuracy and verifiability.
MCA
(Measure Cognition and Action)
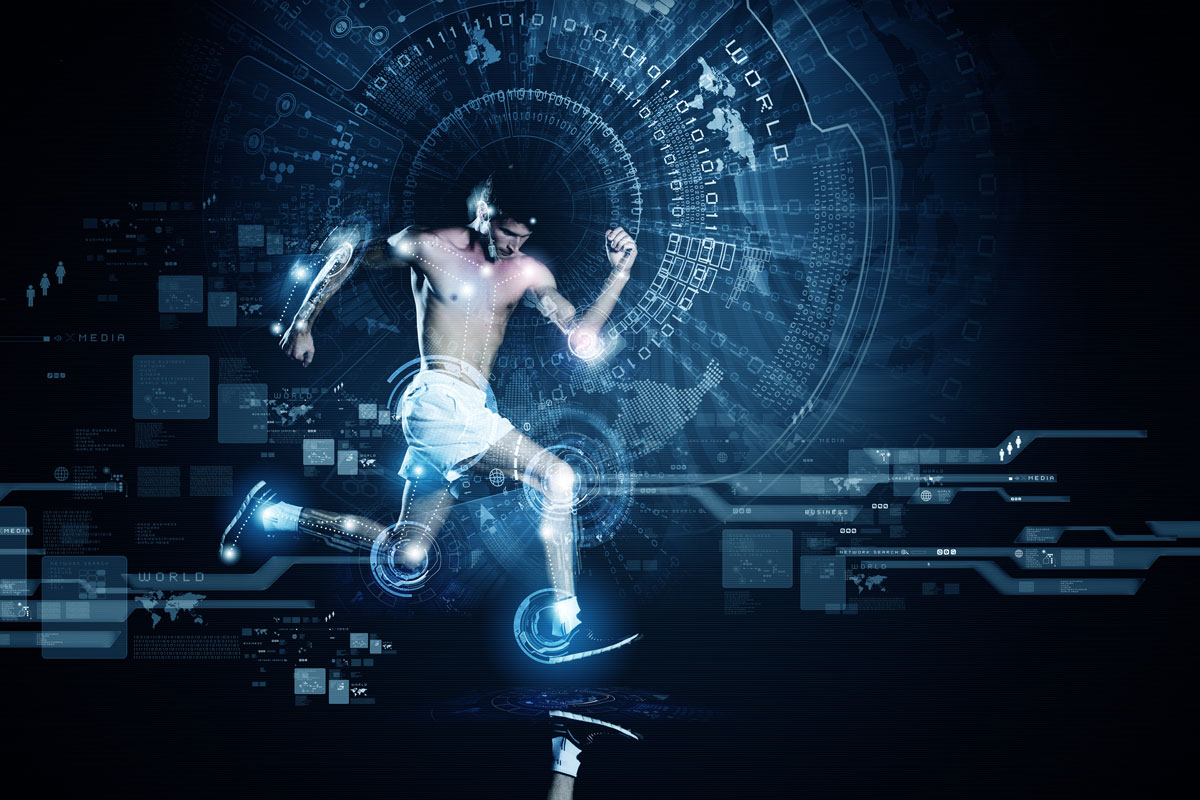
Its a tool developed together with our partners COGNITIVE-MOTOR PERFORMANCE SPA., which applies evaluation and training methods to optimize the cognitive-motor skills of athletes.
Thanks to our cutting-edge technology, we can help managers and coaches of open-structured sports clubs by evaluating and training cognitive-motor skills, to promote the effectiveness of athletes’ responses, and in turn, reduce their chances of injury.
The MCA (Cognition and Action Measurement) is the only global technological solution that quantifies and trains cognitive-motor speed. Our software, through a screen, provides users with sequences of visual stimuli equivalent to competitive situations, in which the athlete must make the best decision (attack or defend), and then move on the contact surface.
The measurement system involves a camera that determines different response times and percentage success/error rate for each evaluation and/or training sequence.
How can sports performance be improved?
Today it is essential to optimize the processes of perception, decision making and motor execution. The correct integration of these allows the athlete to respond satisfactorily to the innumerable, varied and uncertain situations that occur in competition under time limitations.
For this reason, the need arises to design and implement evaluative methods that make it possible to obtain integrated quantitative information representative of the cognitive-motor performance of athletes, and based on this, structure specialized training programs to improve cognitive-motor speed.
Images
Video
Intelligent Sensing System for the
Agro-Fruit Industry – (SIAG)
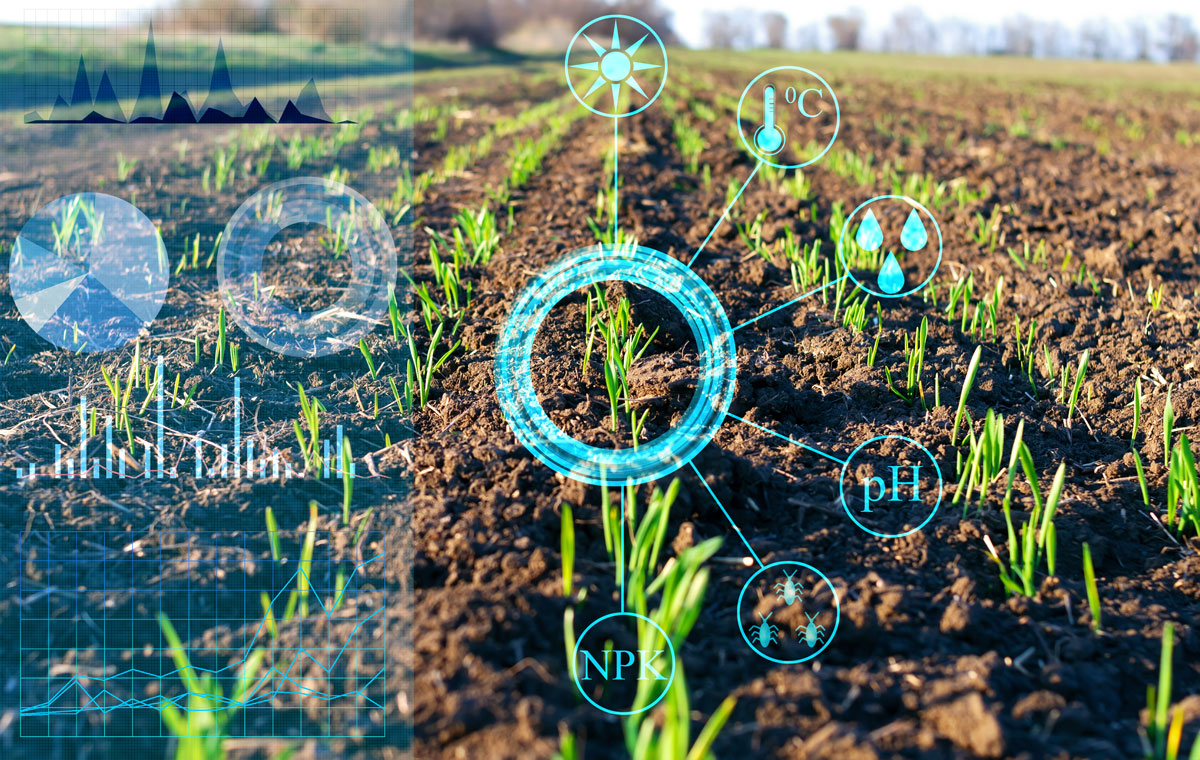
Wildsense Ltda. develops an IOT sensing system to assist in data extraction and relevant information in critical processes related to agricultural cultivation, specifically in fruit production.
These processes include being able to monitor the performance and behavior of the harvesters, being able to determine the ripening and quality of the fruits, making production projections and sensorizing the pressure in the different irrigation sources, the aim is to implement a system in the industry modular, which allows the various associated services to be packaged, in order to provide a service adjustable to the needs of different properties and clients in a joint piloting study.
Priority projects to be developed.
Follow-up of harvesters.
It is desired to monitor the harvesters in order to capture information, such as: their trajectory during the day, how much fruit the worker harvested and their health status during the work day, among others.
For this reason, Wildsense proposes the use of a low-cost smart band or bracelet, which will be responsible for transmitting all this information to a central system, for processing and subsequent analysis.
Processing of images, videos of ripening and fruit quality.
A mechanism for sensing and integrating information, fast and efficient, is sought to know the ripening and quality of the fruits.
This factor is extremely important when it comes to production, which is why Wildsense will implement, together with a network of sensors and cameras distributed throughout the plantations, artificial intelligence components capable of identifying quality and ripening criteria in relation to the different fruits that are presented, in order to be able to evaluate the performance of the production process online.
Data analysis and projections for decision making.
It seeks to analyze all the information collected by the network of sensors, cameras, GPS, ripening and quality of the fruits and the work of the harvesters, among other characteristics, in order to make projections and estimates of production in future months of work.
To do this, all the components of the system will be connected to the main class, which will carry out these analyses, estimates and send them through different channels so that they can later be viewed in the different applications.
Some of these are being able to visualize how the plantations are progressing or the work carried out by a harvester, among others.
Sensorization to obtain the pressure of irrigation canals.
A problem that arises in the irrigation of plantations is the water pressure, which differs between origin and destination, which implies loss of control in the cultivation process and the misuse of water resources.
For this reason, a control and sensing system of the pressure of the irrigation canals is proposed.
Solution.
A multimodal IoT sensing system is proposed to extract and integrate relevant information in critical processes associated with agricultural cultivation, with emphasis on fruit production.
The system must be able to solve the aforementioned problems. It is worth mentioning that due to the size of the crops and the technology that will be used, it is impossible to connect the entire network of sensors, cameras and GPS directly to the main module of the system.
This is why it was decided to separate the fruit growing fields into different sections in the shape of hexagonal cells, as can be seen in the following image.
This allows a better distribution of the different sensors since there are stationary sensors, as mentioned above, and other mobile ones. Each sector will have a “Base Station”, which will be in charge of connecting to all the sensors inside the cell and collecting the information that each sensory component delivers.
Finally, the main module, located in the Core Layer, will be in charge of connecting with each base station in order to collect the information of all the crops present. The WildSense architecture and its issues are described in more detail below.
System Architecture.
Since the sensor network uses different access technologies, a system is proposed where the access layer has data reading interfaces, specially designed for each type of network or communication protocol used by each sensing component. In the following diagram at least 3 access technologies are mentioned, MAVLINK for drones, WiFi and LoRaWAN for the rest of the sensors.
LoRa Network Architecture
The size of the land is highly variable from hundreds of meters to kilometers in length. This fundamentally depends on the type of fruit that is harvested and the geographical location.
For example, the fruit fields in Chile are much smaller than those in Peru. Therefore, the choice of network technology becomes a challenge. As a result, it is proposed to use LoRaWAN for the network, because this new communication technology has been created for Low Power Wide Area Network (LPWAN) and it is relatively cheap to cover a new area with devices compatible with this technology.
The communication range has become the critical part in most IoT systems and with the appearance of this technology, more than km can be reached in rural areas with very low power consumption.
LoRa covers the physical layer for wireless communication, while LoRaWAN establishes the communication protocols and network architecture which determine the lifetime of nodes, network capacity, quality of service, security, and wide variety of applications supported by the network.
LoRa is capable of managing many nodes in multiple locations within the area, unlike Wi-Fi based systems for example, which need to have many access points to increase the coverage area.
The combination of LoRa and WiFi technology reduces the cost of IoT system deployment. In addition, LoRa uses the Industrial, Scientific and Medical (ISM) frequency bands that do not require a license to use, which accelerates the deployment and implementation of the network.
The figure below shows the general architecture of a LoRa network which is made up of four fundamental elements: LoRa nodes, gateways, network server and application servers.
..
General architecture of a LoRa network.
If an analogy is made with our scenario, the LoRa nodes would be the bands/wristbands capable of transmitting all the necessary information to the gateways using a chirp spread spectrum (CSS) modulation as defined in the LoRa physical layer.
This type of modulation is very efficient in terms of energy consumption, similar to the frequency shift keying (FSK) modulation used in most wireless communications, but it also allows reaching great distances.
The gateways must be properly deployed on the premises and are intended to receive messages from all nodes in their coverage area and forward them to the network server.
The connection between the gateways and the network server is made through a high-bandwidth network such as WiFi, Ethernet or cellular (3G/4G), unlike the connection between the nodes and the gateway that is carried out through the LoRaWAN communication protocol. On the other hand, the network server is in charge of routing the messages coming from the nodes to the specific application and vice versa.
Lastly, the application servers will have specific tasks depending on the requirements of the project. For example, one of them will be in charge of processing the signals coming from the bands/bracelets to determine the position of the harvesters at each instant of time.
The number of elements that make up the network and the best way to distribute them depends on the work scenario, the number of tasks that are going to be executed in the network and the precision that is to be achieved.
Each harvester would wear a band/bracelet representing the mobile nodes. For simplicity, ChirpStack (https://www.chirpstack.io/) was chosen as the network server and there will be an application server associated with each of the specific objectives of the project.
The gateways that must be deployed on the premises to guarantee the performance of the multimodal system depend on the desired precision in the location of the nodes and, therefore, will be closely related to the implemented algorithm.
Location in LoRa networks.
The location techniques applied to LoRa networks follow the same principles used in traditional wireless networks. Firstly, a Global Positioning System (GPS) could be used to determine the position of each harvester on the farm, although this is not always feasible because trees can limit coverage and, more importantly, significant energy consumption is required. for a wearable device like a band/wristband.
As a consequence, it is proposed to use other techniques that dispense with GPS, which are divided into range-based or range-free. Rank-free solutions are used to estimate the behavior of groups of nodes and are therefore not suitable for accurately tracking each harvester.
On the other hand, range-based solutions are the most feasible, since they are based on determining the position of the target node with respect to other reference nodes, through different techniques such as: Time of Arrival (ToA), Time Difference of Arrival (TDoA), Received Signal Strength Indicator (RSSI) and Angle of Arrival (AoA).
Solutions based on the RSSI parameter are the simplest to apply, since it is only necessary to estimate the power of the received signal assuming the transmission power is known. Thus, the distance traveled by the signal depends on the propagation model that best fits the work scenario.
Having the distance from each harvester to one or more reference nodes (gateways) it is possible to form circles with center in the node to locate and the radii will represent the previously calculated distances. The position of the harvester is determined through the intersection of these circumferences, which is known as trilateration or multilateration.
This solution has the disadvantage that it is not easy to accurately determine the relationship between the attenuation of the signal power as a function of the distance traveled and, therefore, a location error would be incurred.
On the other hand, solutions based on AoA are more complex to implement because the angle of arrival of the signal must be obtained, which is possible using a multiple antenna system.
Using or more gateways, lines are drawn with the appropriate angle and the intersection represents the position of the harvester, which is known as triangulation. The disadvantage of this method lies in the complexity of the receiver design and the processing of the received signal.
The operating principle of the ToA technique consists of calculating the time it takes for the signal to propagate from the band/wristband to one or more gateways. With each time and knowing the speed of propagation of an electromagnetic wave in the air (m/s), the relative distance between each harvester and the corresponding gateway can be calculated. The last step is to apply trilateration to determine the position of each harvester.
The main disadvantage lies in guaranteeing a perfect synchronization between the transmitter and the receiver, to accurately calculate the time of flight of the signal. For example, a time measurement error of ns is equivalent to a distance of m.
The TDoA method is based on calculating the difference in the propagation time of the signal from the band/wristband to each gateway. With this time difference, the difference in distances can be calculated, which then allows the construction of a series of hyperbolas associated with each difference in distance between the band/bracelet and the corresponding gateway.
The point where or more hyperbolas intersect, corresponds to the position of the harvester. This solution only requires synchronization between the gateways to calculate the difference in arrival time, which is an advantage since LoRa technology defines its own strategies for synchronization. This makes TDoA the most favorable solution to implement for locating and monitoring harvesters on farms.
The figure below represents the proposed model, where the central circle represents a band/wristband with support for LoRa, the three communication towers are equivalent to the minimum number of gateways that, as shown, are complemented by GPS to guarantee synchronization, and the network server routes the messages coming from each gateway to the application server (for example: Geolocation Solver) where the algorithm for harvester location will be implemented.
Proposed model for the positioning of harvesters.
Infrastructure for image processing and data analysis.
There are other objectives that are somehow supported by the network infrastructure that is proposed to be created to determine the location of the harvesters. One of them is the use of complementary technologies for bands/bracelets that make it possible to extend their use to access control, identification and registration of the daily production of each individual. For this task, the Radio-Frequency Identification (RFID) or Near-Field Communication (NFC) connectivity technology is proposed.
Low power consumption is essential because we would have a large number of bands/bracelets and, therefore, the time between each battery recharge should be as long as possible. The way to extend the life of batteries is through energy conservation or energy harvesting techniques.
The first aims to maximize the time or the number of operations that can be carried out with the available energy, and the second complements the operation of the devices with energy sources from the environment, such as the sun or electromagnetic waves, among others.
The way to collect, store and process all the data coming from the sensors for decision making can be addressed with a mobile station.
This mobile station contains a digital scale to deposit the harvested fruits and associate each load with a user via RFID. This solution can include photo/video cameras to monitor the evolution of the fruits in the production process.
Through this method you can have a more accurate record of production, and perform better planning and decision making.
Software Architecture.
There is a 3-layer microservices system:
- Access, where sensing components are accessed through input and output interfaces (APIs).
- Central, where the processing services are.
- User, where are the user applications that require the previous layers.
Images
Automated Subsea Robotics for High Seas Salmon Farming (R-SASA)
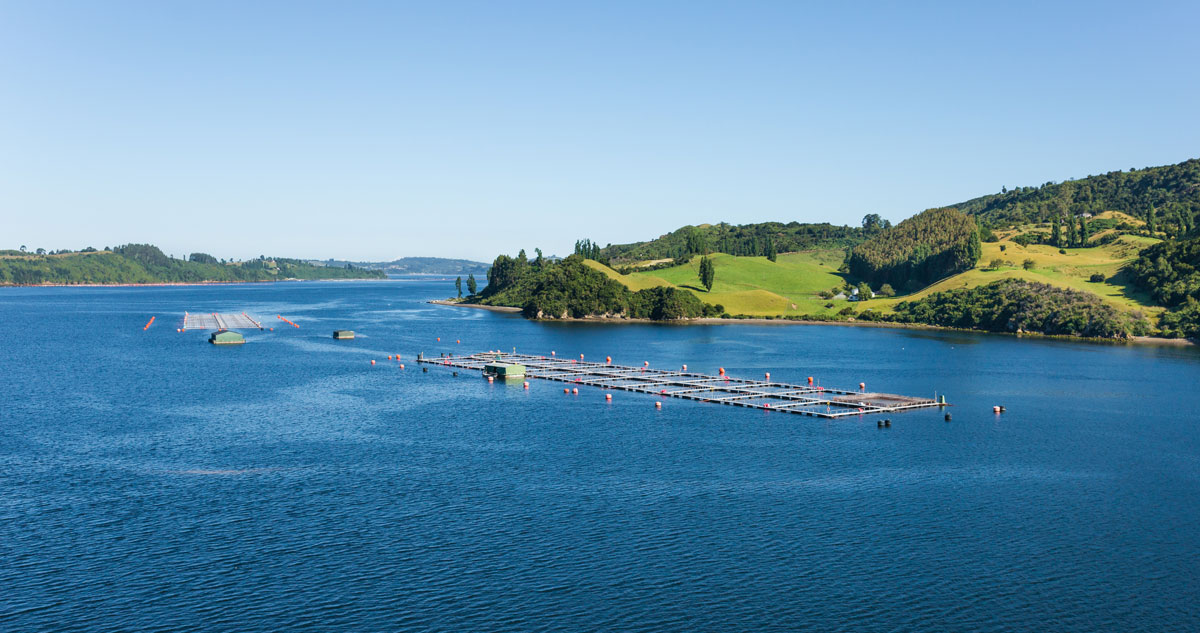
Inspection processes in offshore salmon farming cages currently have low technological sophistication, accuracy and verifiability. These processes are critical to reduce the environmental and social impact of the sector, subject to increasingly demanding regulations.
The salmon sector in Chile is made up of app. 500 cultivation platforms in total. The standard cost of ROV services to each platform is USD74,400/year giving a market potential of USD37.2 million/year (TAM).
Only 13 companies have 50% of these platforms, with a greater capacity to acquire technology, equivalent to a SAM of USD18.6 million/year.
We have created an intelligent IoT System, incorporating robotics and artificial vision to automate ROVs (underwater robots) in the inspection of culture meshes and the monitoring of environmental variables inside the cage.
The platform allows access to relevant information from the system, to improve the decision-making capabilities of the salmon industry and reduce its impact.
Currently the R-SASA System applies the following operational solutions:
- ROV positioning system with ultrasound.
- Fouling detector (algae dirt), with accuracy greater than 80%, using artificial vision.
- Mesh breakage detector, with accuracy around 95%, using computer vision.
- 3D modeling and visualization of culture cages, incorporating visual information to the inspection process (coverage, breakage and fouling).
- Modular architecture software for data acquisition, processing, analysis and visualization.
- Refinement and calibration of ROV positioning systems.
- Refinement of artificial vision algorithms for breakage and fouling detection.
- Integration of devices for measuring environmental variables to the ROV, for characterization of the internal environment of the cages.
- IoT platform debugging, for data integration, transmission and storage, inspection visualization and generation of dashboards and reports for analysis.
- Detection of algae accumulation in chop meshes.
- Biomass estimation with stereo depth analysis and artificial vision.
- Identification of wounds by Caligus and SRS.
Images
Videos